Cybersecurity and AI/ML, Before The New Age of AI: Endpoint Security
Preventing endpoint infection with full-featured AI/ML-powered endpoint detection and response (EDR) solutions.
The Age of AI is upon us. While that entails multiple positive leaps in the evolution of systems, processes and productivity, cybersecurity threats have evolved as well. This is Part I of a six-part series by Derek E. Brink of Aberdeen Strategy & Research. In this article, he unpacks the latest research by Aberdeen on how AI/ML-powered endpoint detection and response (EDR) solutions are more capable of preventing and fighting back evolved endpoint infection attacks than traditional approaches to endpoint security.
My sense is that most people today agree with Bill Gates, who wrote on July 11, 2023, that “we are now in the earliest stage of another profound change, the Age of AI.” Never mind that the technical roots of artificial intelligence (AI) and machine learning (ML) go back decades — in today’s public square, the big bang event for the Age of AI took place on November 30, 2022, with the introduction of ChatGPT. Within just two months, ChatGPT reached 100M users, and within another six months, it seems everyone is scrambling to come to grips with the possibilities — both good and bad — of yet another “What hath God wrought” moment in high tech history.
Evolving Endpoint Security Solutions and How To Leverage Them
In cybersecurity, leading solution providers have, in fact, been leveraging AI/ML for several years prior to this top-of-mind timeline. For example, in July 2021, Aberdeen wrote about Quantifying the Risk Reduction of Evolving Endpoint Security Technologies, which described how:
- Traditional approaches to endpoint security — i.e., anti-virus / anti-malware solutions based on signature updates for known malware files, heuristic file scanning, and static analysis — are highly effective, but for a rapidly shrinking use case. The problem is that threat actors are moving aggressively towards polymorphic malware designed to evade traditional signature-based approaches by undergoing multiple permutations and file-less attacks that use existing, legitimate files to compromise a system, making signature-based approaches irrelevant. By themselves, these traditional approaches are no longer enough to reduce the likelihood of a compromise to an acceptable level.
- Dynamic, behavior-based EDR solutions, driven by AI and machine learning, are highly effective — and for a much tougher problem. EDR’s post-execution capabilities go beyond traditional signatures to detect malware that has not previously been seen and provide a range of approaches to defuse it — including the ability to stop processes, block or delete files, and remotely isolate or shut down affected devices.
More specifically, Aberdeen’s quantitative analysis estimated the median likelihood of endpoint infection at that time for each of four common groupings of endpoint security capabilities that are designed to reduce the likelihood of a successful compromise:
- Traditional signatures: 8.5% (or, if you prefer, 91.5% effective)
- Signatures, device discovery, and pre-emptive device posture: 4.7%
- Signatures, device discovery and posture, and virtual patching: 3.7%
- Signatures, device discovery and posture, virtual patching, and dynamic endpoint detection and response: 0.4%
Full-featured EDR Reduces the Median Likelihood of Endpoint Infection by 95%
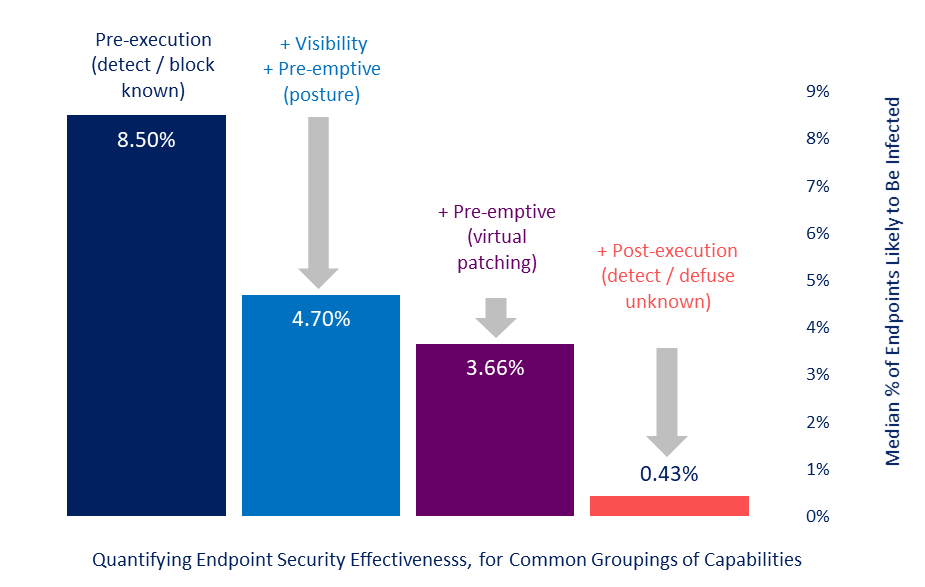
Source: Monte Carlo analysis; Aberdeen, July 2021
Said another way: Aberdeen’s quantitative analysis found that compared to a signatures-only approach to endpoint security, a full-featured endpoint detection and response (EDR) solution — driven by AI/ML capabilities — reduces the likelihood of an endpoint infection by about 95%.
Note that Endpoint Security is just one of several examples — which will be the subject of a series of upcoming articles, so stay tuned — of the natural fit between cybersecurity and three well-known use cases for AI/ML:
- Pattern recognition (illustrative example: filter out noise, “minimize false positives”, free up cybersecurity analysts to work on the most relevant threats)
- Process automation (illustrative example: analyze and correlate data from multiple sources; enable faster triage, forensics, and incident response)
- Predictions (illustrative example: automatically scan networks and systems for vulnerabilities; identify weaknesses most likely to be exploited by attackers; prioritize and recommend patches, updates, or other countermeasures)
In addition, Aberdeen has launched a new research study on AI in the Enterprise: The State of AI in 2023, which will look at AI use cases in several business areas, including Cybersecurity, IT Infrastructure, Sales & Marketing, Financial Management & ERP, Manufacturing & Engineering, and Retail. We’d welcome your input — to participate, you can respond to our survey here.
How are you battling endpoint infection? Does your EDR solution leverage AI/ML? Share your experience with us on Facebook, Twitter, and LinkedIn. We’d love to hear from you!