5 Ways Enterprises Can Make the Most of Their AI Investments in 2022
While organizations have started implementing AI, how can they increase their ROI from the technology-driven initiatives? Discover a few ways to do it.
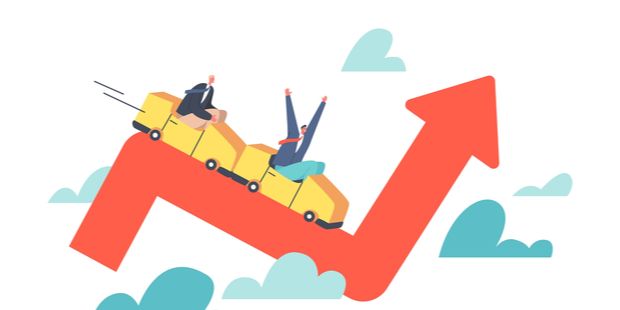
AI has gained more prominence since the pandemic. While organizations have started implementing the technology, how can they increase their ROI from AI-driven initiatives? Sunil Senan, Senior Vice President Business Head – Data & Analytics, Infosys explains a few ways to do it.
Artificial Intelligence (AI) has been enjoying a bull run since the pandemic. One estimate says that the AI market’s global revenues will cross US 327 billion this year and grow at a CAGR of 17.5% to cross US$ 550 billion by 2024. Another source predicts that 70% of organizations will likely adopt at least one AI technology by 2030.
Although AI has become a fundamental capability that has started to permeate organizations, businesses struggle to understand and focus on the initiatives that will deliver the most value. Inability to understand the impact of AI on their economics, lack of a strategic framework to prioritize initiatives, scarcity of AI-engineering knowledge and talent are just some deterrents.
So, what can we do to increase our return on investment from AI-driven initiatives?
1. Establish a Strategic Framework To Identify and Fund Programs
AI as a technology alters the economics of prediction. To deliver value, however, these predictions need to be acted on appropriately. Predictions are subject to human judgment and a decision framework to arrive at the actions to be taken. The actions and the resulting outcomes are what produce business value.
Companies need to adopt a strategic framework to understand business impact and prioritize investments in AI initiatives. An AI canvas like the Merge framework can provide the strategic lens to look at predictions using AI, judgments to be made, actions that create results and the outcomes. This acts as a human amplifier to decide on the best business processes that could benefit from the use of AI and produce maximum business value.
AI initiatives are continuous processes that leverage relevant data to make predictions; the outcomes from these predictions provide the necessary feedback to improve the prediction models, with systems learning from this data. The new learning may also lead to the use of additional data for the prediction process. This process continues until the required accuracy of prediction is achieved.
See More: Maintenance Staffing Broken? AI May Be the Fix You Need
2. Experiment To Industrialize
Implementing AI initiatives is not a linear path. It starts with gathering the appropriate data and conducting experiments to decide on the right model or ensemble of models for the prediction. The results of the experiment need to be recorded and used to learn from.
The industrialization of AI is a challenge for most enterprises. This is one of the reasons for many AI initiatives not evolving into production. Automating this process using MLOps pipelines can prove useful. This includes everything from teaching the model, testing the model, deploying it and monitoring it to make sure that it is functioning correctly without bias and other data-related complications.
Companies also have success when they adopt a foundry and factory approach to experiment and industrialize AI solutions.
3. Embrace a Micro-change Management Framework
The adoption of AI prediction in the process framework is a significant change management process. Human prediction capabilities are sub-planted by AI prediction models, and the emphasis for the human actors will lean towards judgment and action. This needs to be addressed and managed. A micro-change management framework that realizes this change in small measurable steps allowing the overall system to adapt to this significant change effectively can prove invaluable.
See More: A Call for Governance and Responsibility in AI
4. Leverage the Partner Ecosystem
Powerful trends, such as the open economy, platformification of business, and embedded experiences, are all pointing enterprises towards their ecosystems. Organizations must lean on their partner networks and wider ecosystems to maximize the value of their AI investments. For example, businesses need more people in more roles to extend AI to various stakeholders; since skills are scarce, they can work with external developers to build and innovate new solutions.
By building joint ventures with partners, including other firms, enterprises can pool their data to create the large data sets required to train machine learning algorithms. At some point, the venture could also pool financial resources to spur in-house innovation or create a venture capital fund to invest in start-ups with disruptive AI solutions. This would also give organizations immediate access to the start-ups’ customers. The most successful companies on the planet have all gone down the acquisition route: between them, Amazon, Apple, Facebook, Google and Microsoft made 13 AI acquisitions in 2020.
Enterprises can tie up with adjacent businesses to embed their AI solutions within their partners’ customer journeys to reach a much wider audience. For illustration, look no further than Google Maps.
An emerging aspect of leveraging the partner ecosystem is leveraging learning from the action and outcomes of AI initiatives. This is extremely valuable data, and enterprises often look to monetize it. The secure sharing and management of this data is key for the industrialization of AI initiatives.
5. Scale AI-readiness in the Workforce
AI is becoming a fundamental capability. With it, there is a growing need to nurture full-stack engineers who are equipped to work with AI. This is key to scaling AI. Unfortunately, skills and know-how continue to be in short supply. A part of this need is filled by easy-to-use tools and the creation of citizen data scientists. These are analysts armed with tools who can perform most of the common functions of a data scientist. However, there is also a need for companies to create programs for creating full-stack engineers with foundational AI knowledge to execute and operationalize AI initiatives.
See More: Unlock Stronger Customer Relationships With Natural Language Processing
Since the AI skills gap will only widen, enterprises need a strategy for feeding their future talent pipelines. One option is to expose college and even school students to AI learning through the means of online tools such as Coursera, Udemy, Udacity, and edX.
A difficult but important step is to change the organization’s culture to emphasize lifelong learning. AI skilling is not a one-off activity. To be meaningful, it must be offered regularly and repeatedly throughout an employee’s career. Training should cover not only the latest technological advances but also the surrounding higher-order skills, like creative thinking, ethics, and problem-solving, that are essential for succeeding at AI.
Did you find this article helpful? Tell us what you think on LinkedIn, Twitter, or Facebook. We’d be thrilled to hear from you.