How Big Data, Analytics and ML Can Transform Your Insurance Business
The increased use of big data, analysis and machine learning has the capability to transform the way insurers do business.
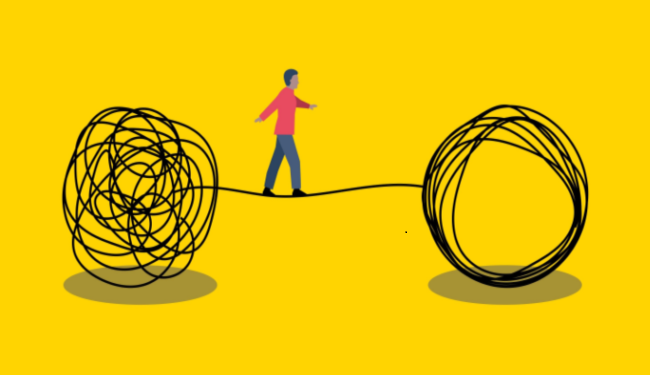
Enterprises require big data capabilities, machine learning and the ability to bring all the analytics together and deliver it to those who will benefit most, discusses Vinod Singh, CTO, Concirrus.
The increased use of big data, analysis and machine learning has the capability to transform the way insurers do business. However, there are three separate parts to this process, and they need to be implemented in harmony within your technology solution to living up to their full potential.
See More: How Graph Analytics Can Transform Enterprise Data Protection
One Step at a Time
We believe that step one is to bring the data together via your data pipelines into a single place we call a data lake. Once you have your data housed, you need the capability to discover and manage that data. In other words, you need to be able to understand what information you have and where it is stored within the lake. That will lead you to create a data catalog, which should be accompanied by the ability to understand the lineage. This requires an understanding of where the data has come from, the source, the quality, and what, if anything, has been done to it.
The data lake can then feed into the machine learning and modeling stack, which delivers the business insights you need to the right people. You cannot build the value for the business until you have all three elements in place. For example, you can have a big data platform that can store all the information in the universe but without machine learning, the data is still essentially unusable as it cannot be analyzed. In addition, you may have the best big data and machine learning functions, but unless you can deliver the right data to the right people at the right time, it’s unlikely to function the way you want it to and, in turn, will not deliver the business benefits you seek.
Big Data Delivers Multiple Choices
When it comes to the big data stack, I would say there are more than two hundred options. Even if you simply focus on those seen as the dominant players, there are still around 50.
It is vital to consider how you will need to access your data. Will it be in bulk or is it very much a requirement to access specific data for specific processes and uses? Will the data be streamed constantly, or is it the case that it arrives and is updated monthly? You need to dig deep into the type of data you are looking to manage and exploit.
How stringent are your access needs? Will confidential data be stored, and will there be a requirement for access to be strictly controlled in terms of who can access what? Does the data need to be accessed in real-time, or is it sufficient for the data to be accessed after an hour?
Invest in Planning ML Requirements
When it comes to machine learning, there are many dominant platforms across a range of tasks. For example, you may wish to use the deep learning systems to build a predictive risk model as part of the platform, however, this requires a huge amount of data, which may necessitate data from hundreds of millions of claims which is simply unavailable.
You can quickly determine that by looking to solve one problem, you are simply creating another. You need to be clear on the type and scale of the advice you are looking to generate. Once that has been agreed upon, then it goes back to the data. You will need to look at how you can link your preferred output with the data.
You have two very distinct types of systems. The first is best described as an open and transparent bespoke system that requires a good deal of work to integrate with your current operations. The second is the commercially available technology, which is your ‘black box’ system that can be rapidly integrated but may not have the complete functionality you need.
Ideally, you do not want to be at one end of either system option but instead, have a mixture of both.
Delivery of Analytics
With the first two in place, you need to decide how you want to deliver the analytics and to whom. Is it to developers, and if so, do you need an API? Are they unsophisticated users, and if so, do you need a clear, user-friendly dashboard?
The platform must understand your business and the needs of the individual departments, be they underwriting or claims, and speak the business’ language.
A common mistake businesses make is once all the data challenges have been solved, companies will undertake a small proof of concept, find that the machine learning works and immediately assume it will work across the business. But all too often, this is not the case. It will take time and effort to realize the benefits of machine learning across your organization.
See More: How Learning Analytics Blueprint Helps Organizations Future-proof Operations
Is there a Best Way Forward?
Your investment in technology does depend on the maturity of the business. In theory, you would want to automate from end to end across the business and do it from day one.
That would be best practice for a mature business with a clear idea of what it wants and what it will look like and do it right first. But if you have a less mature business, the best practice would be to start small with a mixture of manual and automation. As your business develops and you have a better sense of your requirements, you can increase the level of automation in the tasks where you can clearly understand the benefits it will bring.
Design and implement for future needs but do not try and design and implement for future unknowns. Look to continuous delivery of small parts of the plan. It allows the system to change as and when requirements change.
How is your business benefiting from Big Data, analytics and ML? Share with us on Facebook, Twitter, and LinkedIn. We’re always eager to hear from you!